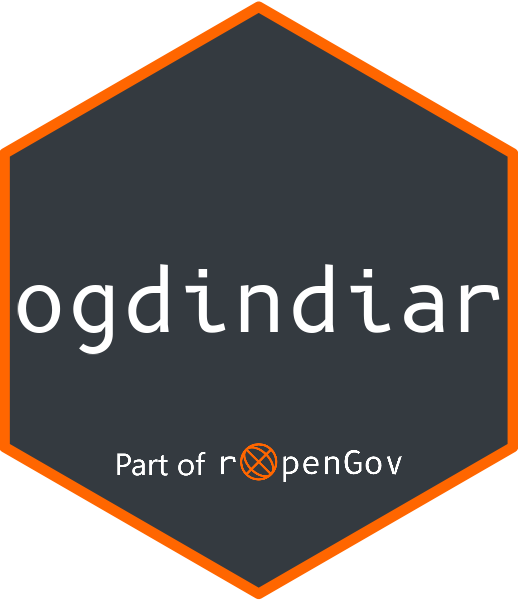
Introduction
Dhrumin Shah
2015-08-22
Source:vignettes/basic-usage-vignette.Rmd
basic-usage-vignette.Rmd
Introduction
This package provides easy access to the API provided by Open Government Data Platform - India to download datasets from R. Here’s the list of Datasets available through API.
Basic Usage
## Welcome to ogdindiar
When calling OGD India API, at minimum, you need to provide 2 parameters.
-
res_id
: Resource id of the dataset you want to access -
api_key
: Your personal API key (See Package Installation instructions)
Resource id for the datasets can be found on the data specific page on the data portal. For example, this page has the resource id information for Annual And Seasonal Mean Temperature Of India. The resource is a string that’s part of Datastore API URL.
- The URL for this dataset as shown on the page is - https://data.gov.in/api/datastore/resource.json?resource_id=98fe9271-a59d-4834-b05b-fd5ddb94ac01&api-key=OGDINDIA_API_KEY
- The resource id is the highlighted part in the above URL.
The main function this package provides is fetch_data()
.
Once you have figured out the resource id, you can download that dataset
as follows:
mean_temp_data = fetch_data(res_id = "98fe9271-a59d-4834-b05b-fd5ddb94ac01")
This function returns a list of 2 elements.
- The first element is the data
id | timestamp | year | annual | jan_feb | mar_may | jun_sep | oct_dec |
---|---|---|---|---|---|---|---|
1123 | 1424778424 | 1957 | 23 | 18 | 25 | 27 | 21 |
1423 | 1424778424 | 1972 | 24 | 18 | 25 | 27 | 21 |
1443 | 1424778424 | 1973 | 24 | 19 | 26 | 27 | 21 |
1463 | 1424778424 | 1974 | 24 | 18 | 26 | 27 | 21 |
1483 | 1424778424 | 1975 | 23 | 18 | 25 | 26 | 21 |
1503 | 1424778424 | 1976 | 24 | 18 | 25 | 26 | 22 |
- The second element is a dataframe containing metadata about the columns.
knitr::kable(mean_temp_data[[2]])
.id | type | size | unsigned | not null | description |
---|---|---|---|---|---|
id | serial | normal | TRUE | TRUE | |
timestamp | int | normal | TRUE | FALSE | The Unix timestamp for the data. |
year | int | normal | NA | FALSE | |
annual | int | normal | NA | FALSE | |
jan_feb | int | normal | NA | FALSE | |
mar_may | int | normal | NA | FALSE | |
jun_sep | int | normal | NA | FALSE | |
oct_dec | int | normal | NA | FALSE |
Advanced Usage
Instead of downloading entire datasets you can conditionally download
specific data elements. This functionality is achieved using additional
arguments to fetch_data()
function. Currently you can use
-
-
filter
to filter the dataset using equality constraints on specific columns. -
select
to select specific set of columns to be downloaded. -
sort
to sort the resulting dataset based on multiple columns.
Following example illustrates this -
mean_temp_25 = fetch_data(res_id = "98fe9271-a59d-4834-b05b-fd5ddb94ac01",
filter = c("annual" = "25"),
select = c("year", "annual", "jan_feb", "mar_may", "jun_sep", "oct_dec"),
sort = c("jan_feb" = "asc", "mar_may" = "desc")
)
The returned dataset -
year | annual | jan_feb | mar_may | jun_sep | oct_dec |
---|---|---|---|---|---|
2002 | 25 | 19 | 27 | 27 | 22 |
2010 | 25 | 20 | 27 | 27 | 22 |
1995 | 25 | 20 | 26 | 28 | 23 |
2009 | 25 | 20 | 26 | 27 | 22 |
2006 | 25 | 21 | 26 | 27 | 22 |
Metadata about the returned dataset
knitr::kable(mean_temp_25[[2]])
.id | type | size | not null | description |
---|---|---|---|---|
year | int | normal | FALSE | |
annual | int | normal | FALSE | |
jan_feb | int | normal | FALSE | |
mar_may | int | normal | FALSE | |
jun_sep | int | normal | FALSE | |
oct_dec | int | normal | FALSE |
There’s one more argument that is passed to fetch_data()
function, field_type_correction
. The data fetch process
inadvertently treats all the columns as character
. The
default setting field_type_correction = TRUE
converts these
columns back to numeric
type based on accompanying
metadata.